summary: Using neuroimaging data, a new deep learning algorithm was able to detect Alzheimer’s disease with 90.2% accuracy.
sauce: Mass in general
While researchers have made progress in detecting signs of Alzheimer’s disease using high-quality brain imaging tests collected as part of their study, a team at Massachusetts General Hospital (MGH) recently developed an accurate detection method that relies on clinical brain images collected in . This advance could lead to more accurate diagnoses.
About the research published in pro swanMatthew Leming, Ph.D., research fellow at MGH’s Center for Systems Biology and investigator at the Alzheimer’s Disease Research Center in Massachusetts, and his colleagues are working on a form of machine learning and artificial intelligence that uses large amounts of data and complex algorithms. I used some deep learning. Train your model.
In this case, scientists developed a model for Alzheimer’s disease detection based on data from magnetic resonance imaging (MRI) acquired from patients with and without Alzheimer’s disease seen on MGH before 2019. .
The group then tested the model on five datasets (MGH since 2019, Brigham and Women’s Hospital around 2019, external system around 2019) to accurately detect Alzheimer’s disease based on real-world data. I checked if it is possible. Global clinical data regardless of hospital or time.
Overall, the study included 11,103 images from 2,348 patients at risk for Alzheimer’s disease and 26,892 images from 8,456 patients without Alzheimer’s disease. In all five datasets, the model detected Alzheimer’s disease risk with 90.2% accuracy.
Among the main innovations of this study was the ability to detect Alzheimer’s disease regardless of other variables such as age. “Since Alzheimer’s disease usually develops in older people, deep learning models often have difficulty detecting rare early-onset cases,” he said.
“We addressed this issue by ‘blinding’ the deep learning model to brain features that were found to be overly associated with the patient’s documented age. “
Leming points out that another common challenge in disease detection, especially in real-world settings, is working with data that differs significantly from the training set. For example, a deep learning model trained on his MRIs from General Electric scanners may not recognize MRIs acquired on Siemens scanners.
The model used an uncertainty metric to determine if patient data were too different from what it was trained to make a successful prediction.
“This is one of the only studies that has attempted to detect dementia using routinely collected brain MRIs. But this study is an important step toward actually doing this in a real clinical setting, as opposed to a perfect lab setting,” said Leming.
“Our results, with their cross-site, cross-time and cross-population generalizability, are a strong case for the clinical use of this diagnostic technique.”
Other co-authors include Dr. Sudeshna Das and Dr. Hyungsoon Im.
Funding: This work was supported by the National Institutes of Health and an innovation program funded by the Ministry of Trade, Industry and Energy of the Republic of Korea managed through subcontracting from MGH.
About this Artificial Intelligence and Alzheimer’s Research News
author: bradon chase
sauce: Mass in general
contact: Bradon Chase – Mass General
image: image is public domain
See also
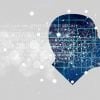
Original research: open access.
“Adversarial confounding regression and uncertainty measures for classifying heterogeneous clinical MRI in Mass General Brigham” by Matthew Lemming et al. pro swan
overview
Adversarial confounding regression and uncertainty measures for classifying heterogeneous clinical MRI in Mass General Brigham
In this work, we introduce a novel deep learning architecture, Multi-Confound Regression Adversarial Network (MUCRAN), to train deep learning models on clinical brain MRI while regressing demographic and technical confounders.
We trained MUCRAN using 17,076 clinical T1 brain axial MRIs collected from Massachusetts General Hospital prior to 2019 and demonstrated that MUCRAN can successfully regress key confounders in a vast clinical dataset. We also applied a method to quantify uncertainty across an ensemble of these models to automatically exclude out-of-distribution data in AD detection.
By combining MUCRAN with uncertainty quantification techniques, newly collected MGH data (since 2019; 84.6% with MUCRAN vs. 72.5% without MUCRAN) and from other hospitals data (90.3%) consistently showed a significant improvement in AD detection accuracy. from Brigham and Women’s Hospital; 81.0% from other hospitals).
MUCRAN provides a generalizable approach for deep learning-based disease detection in heterogeneous clinical data.