AI in medicine is still in its infancy, but we’re already finding racial bias in some tools. Here, medical professionals at a California hospital protesting racial injustice after the murder of George Floyd.
Mark Ralston/AFP via Getty Images
hide caption
toggle caption
Mark Ralston/AFP via Getty Images
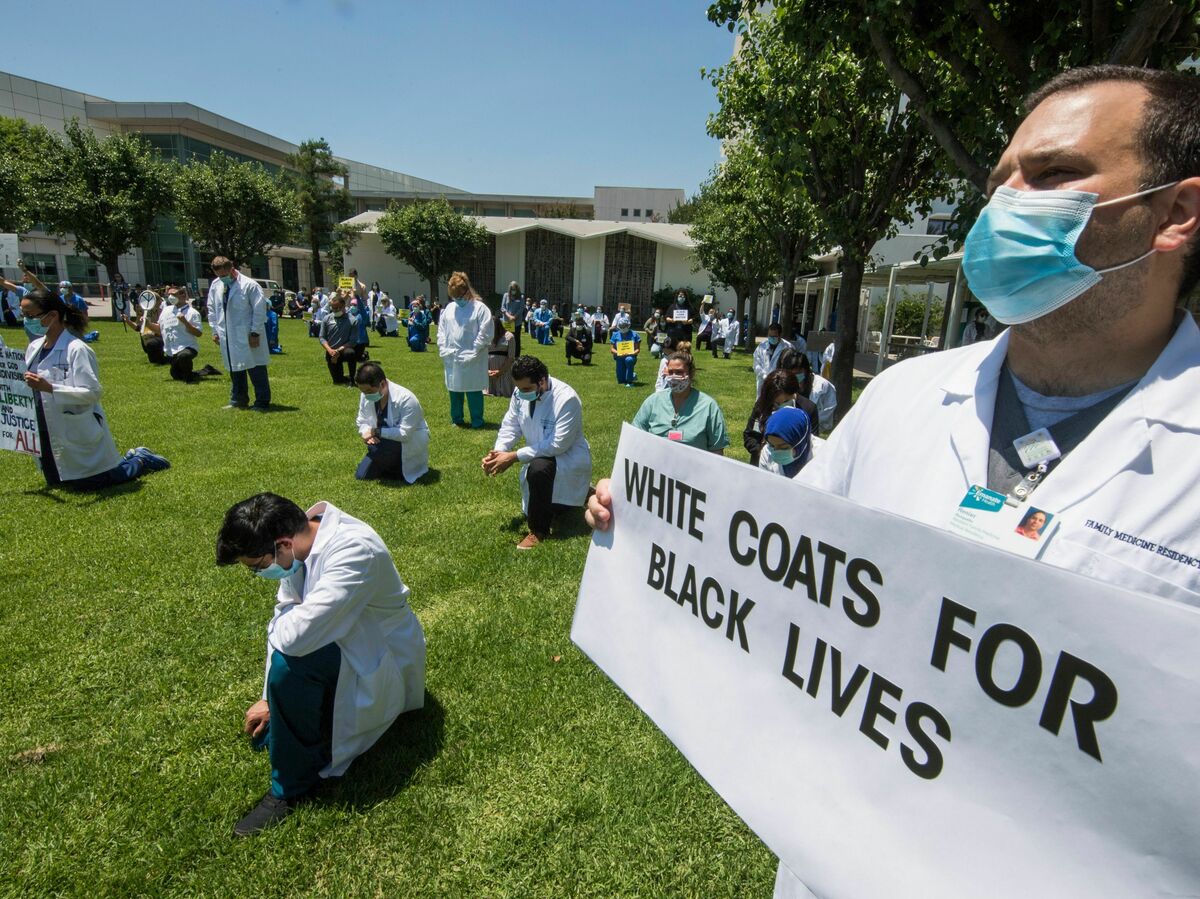
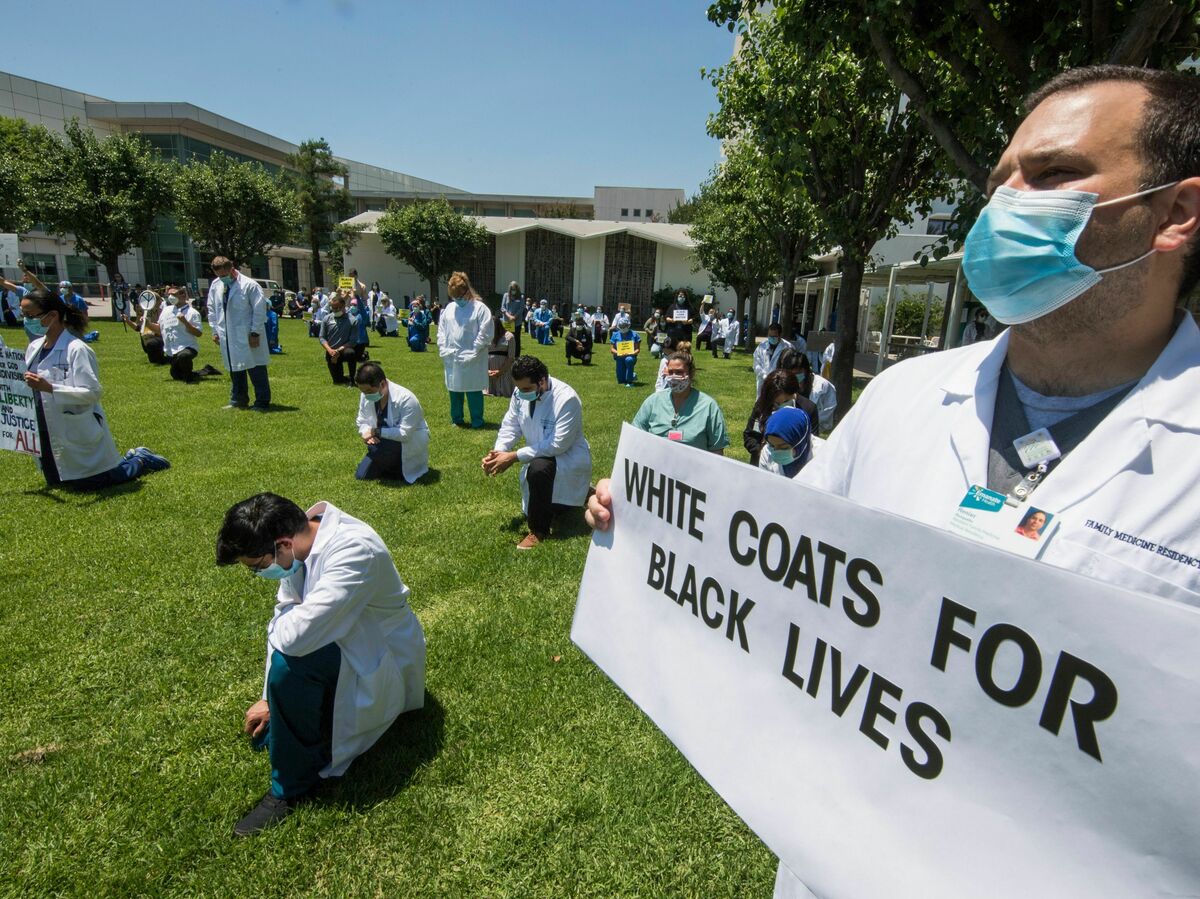
AI in medicine is still in its infancy, but we’re already finding racial bias in some tools. Here, medical professionals at a California hospital protesting racial injustice after the murder of George Floyd.
Mark Ralston/AFP via Getty Images
Doctors, data scientists and hospital executives believe artificial intelligence could help solve previously intractable problems. AI has already shown potential to help clinicians diagnose breast cancer. reading x-rays and Predict which patients need more care. But as the excitement grows, so does the risk. These powerful new tools have the potential to perpetuate longstanding racial inequalities in the way care is delivered.
“If you screw this up, systemic racism can become even more entrenched in the health care system and really, really hurt people,” he said. Mark SendakPrincipal Data Scientist at Duke Health Innovation Lab.
These new healthcare tools are often built using: machine learning, a subset of AI whose algorithms have been trained to find patterns in large datasets such as billing information and test results. These patterns can predict future outcomes, such as a patient’s likelihood of developing sepsis. These algorithms constantly monitor all patients in the hospital at the same time, alerting clinicians to potential risks that overworked staff may miss.
But the data these algorithms are built on often reflects the inequalities and biases that have plagued U.S. healthcare for years.Studies show that clinicians often provide different care Both white patients and people of color. Patient treatment differences are stored in the data and used to train the algorithm. People of color are also common. underrated Within these training datasets.
“When you learn from the past, you are recreating the past. “Because you consider existing inequalities and treat them as aspirations about how health care should be delivered.”
A groundbreaking 2019 study published in the journal Science found that algorithms used to predict The medical needs of over 100 million people are prejudice against black patients. This algorithm predicted future medical needs based on medical spending. However, because of historically less access to treatment, black patients often spent less. As a result, black patients had to get worse before specific treatments could be recommended based on the algorithm.
“It’s essentially walking through a minefield,” Sendak said of attempts to build clinical AI tools with potentially biased data. [if you’re not careful] Your stuff will explode and hurt people. “
The challenge of eradicating racial prejudice
In the fall of 2019, Sendak teamed up with Dr. Donner, a physician in emergency pediatrics. Emily Sterett To develop an algorithm to help predict pediatric sepsis in the emergency department of Duke University Hospital.
sepsis It happens when the body overreacts to an infection and attacks its own organs. Although rare in children, about 75,000 New cases occur in the United States each year. This preventable condition is fatal in nearly 10% of children. Sepsis is effectively treated with antibiotics if detected promptly. However, diagnosis is difficult because typical early symptoms, such as fever, rapid heart rate, and elevated white blood cell count, mimic other illnesses, such as the common cold.
An algorithm that could predict the threat of sepsis in children would be a game changer for doctors across the country. “When a child’s life is at stake, it’s really, really important to have a backup system that AI can provide to compensate for human error,” Sterrett said.
But a groundbreaking study on prejudice, published in the journal Science, underscored the need for Sendak and Sterett to pay attention to design. The team spent a month learning algorithms to identify sepsis based on vital signs and laboratory tests, rather than easily accessible but incomplete claims data. As the program was tweaked during his first 18 months of development, quality control testing was initiated to ensure the algorithm detected sepsis equally regardless of race or ethnicity.
But after nearly three years of deliberate and methodical work, the team discovered that bias could still be creeping in. Dr. Ganga MoorthyA global health researcher at Duke University’s Pediatric Infectious Diseases Program, he said doctors at Duke took more time to order blood tests for Hispanic children who were eventually diagnosed with sepsis than for white children. It showed the investigation of the developer that it took.
“One of my main hypotheses was that doctors were probably taking the illness of white children more seriously than it was of Hispanic children,” Moushy said. She also wondered if the need for an interpreter was slowing the process.
“I was mad at myself. How come I didn’t see this?” Sendak said. “We completely missed all these subtleties. If any of these were true all the time, it could introduce bias into the algorithm.”
Sendak said the team overlooked this delay and may have been giving the AI inaccurate information that Hispanic children developed sepsis later than other children, a time lag that could be fatal. said it could be.
Regulators are also taking notice
Over the past few years, hospitals and researchers have Been formed Nationwide Union share it best practice and develop “Playbook” To fight prejudice. But there are signs that few hospitals are considering the threat to capital this new technology poses.
researcher Paige Non They interviewed staff at 13 academic medical centers last year, and only four said they considered racial bias when developing and scrutinizing machine-learning algorithms.
“If certain leaders in hospitals and health care systems happen to have personal concerns about racial inequality, it will tell you how they think about AI,” Nong said. Stated. “But there was nothing structural. Nothing required at the regulatory or policy level to think or act that way.”
Some experts say that the lack of regulation has left corners of AI feeling like they’re in the “Wild West.” another 2021 investigation We found that FDA policies on racial bias in AI are heterogeneous, and that only a fraction of the algorithms include racial information in public applications.
Over the past 10 months, the Biden administration has released a flurry of proposals for designing guardrails for this emerging technology. FDA says ask the developer now We outline the steps taken to mitigate bias and the data sources underpinning the new algorithm.
National Health Information Technology Coordinating Office Proposing new regulations In April, developers will be asked to share an overview with clinicians about what data was used to build the algorithms. The agency’s chief privacy officer, Catherine Marchesini, described the new regulation as a “nutrition label” to help doctors know “the ingredients used to create the algorithm.” It is hoped that greater transparency will allow healthcare providers to determine whether algorithms are unbiased enough to be safely used with patients.
The U.S. Department of Health and Human Services Office for Civil Rights proposed the latest regulations last summer Explicitly Prohibited for Clinicianshospitals and insurance companies ‘prevent discrimination through the use of clinical algorithms’ [their] decision-making,” said the agency’s director. Melanie Fontes Reiner Federal antidiscrimination laws already ban the activity, but her office said it “certainly [providers and insurers are] Note that this is not just “buy an off-the-shelf product and use it with your eyes closed”. “
Industry welcomes new regulation, but wary at the same time
While many AI and bias experts welcome this newfound attention, there are also concerns. Academics and industry leaders say they want the FDA to spell out in public guidelines exactly what developers must do to prove their AI tools are impartial. rice field. Some have called on ONC to make the algorithm’s “ingredients list” available to its developers so that independent researchers can assess the code for problems.
Several hospital and scholar I am concerned that these proposals, especially HHS’ explicit ban on discriminatory AI use, could backfire. “What we don’t want is for doctors to say, ‘Okay, I’m not using any AI in my practice. I just don’t want to take risks,'” he said. said. Carmel Shachar, Executive Director of the Petrie Fromm Health Law Policy Center at Harvard Law School. Without clear guidance, Shachar and several industry leaders said hospitals with fewer resources could struggle to stay on the right side of the law.
Mark Sendak of Duke University welcomes new regulations to keep bias out of algorithms, “but what regulators haven’t heard is, ‘We’re not going to identify these things. and we understand the resources required to monitor these things.’ We are making investments to ensure that this issue is addressed.”
funded by the federal government $35 billion It is intended to encourage and assist physicians and hospitals to adopt electronic medical records early in this century. The AI and bias regulatory proposals do not include financial incentives or support.
“I have to look in the mirror”
Lacking additional funding and clear regulatory guidelines, AI developers will have to troubleshoot the problem themselves for now.
At Duke University, the team quickly launched a new test after discovering that algorithms that helped predict childhood sepsis may have been biased towards Hispanic patients. It took him eight weeks to finally determine that the algorithm predicted sepsis at the same rate for all patients. Sendak hypothesizes that there were too few cases of sepsis for Hispanic children to be included in the algorithm, causing a delay.
Sendak said the conclusion was more sobering than reassuring. “It’s reassuring that in one particular rare case, we didn’t have to intervene to prevent stigma,” he said. “Every time we discover a potential flaw, we take responsibility for it. [asking]”Where else is this happening?”
Sendak plans to build a more diverse team of anthropologists, sociologists, community members and patients to work together to eradicate the biases of Duke’s algorithm. But for this new breed of tool to do more good than it does harm, Sendak believes the healthcare sector as a whole needs to address its underlying racial inequalities.
“I have to look in the mirror,” he said. “You need to ask tough questions about yourself, the people you work with, and the organization you work for, because if you’re really looking for algorithmic bias, inequality is the root cause of many biases.” Be careful. “